The rapid advancement of artificial intelligence (AI) presents exciting opportunities for innovation and progress. However, it also raises crucial ethical considerations that must be addressed to ensure responsible development and deployment of AI systems. At the heart of these concerns lies the need to foster safe and respectful interactions between humans and AI.
This article will explore key ethical principles guiding the development of AI, focusing on how to create systems that promote safety, respect, and inclusivity. We’ll delve into the importance of avoiding bias and discrimination, ensuring transparency in AI decision-making, and fostering open dialogue about the ethical implications of AI. By understanding these principles, we can work towards building a future where AI technology benefits society while upholding human values.
Ethical AI Principles
Ethical AI development is founded on a set of core principles that guide the design, implementation, and deployment of AI systems. These principles aim to ensure that AI technologies are developed and used responsibly, ethically, and for the benefit of humanity.
Beneficence: This principle emphasizes the importance of designing AI systems that benefit society and promote human well-being. AI should be used to address societal challenges, improve people’s lives, and contribute to a more equitable and sustainable future.
Non-Maleficence: AI systems should be designed to avoid causing harm to individuals or society. This principle requires careful consideration of potential risks and unintended consequences, and the implementation of safeguards to mitigate potential harm.
Autonomy: Individuals should have control over their data and how it is used by AI systems. AI should respect user privacy, provide clear consent mechanisms, and empower users to make informed decisions about their interactions with AI.
Safe Interactions
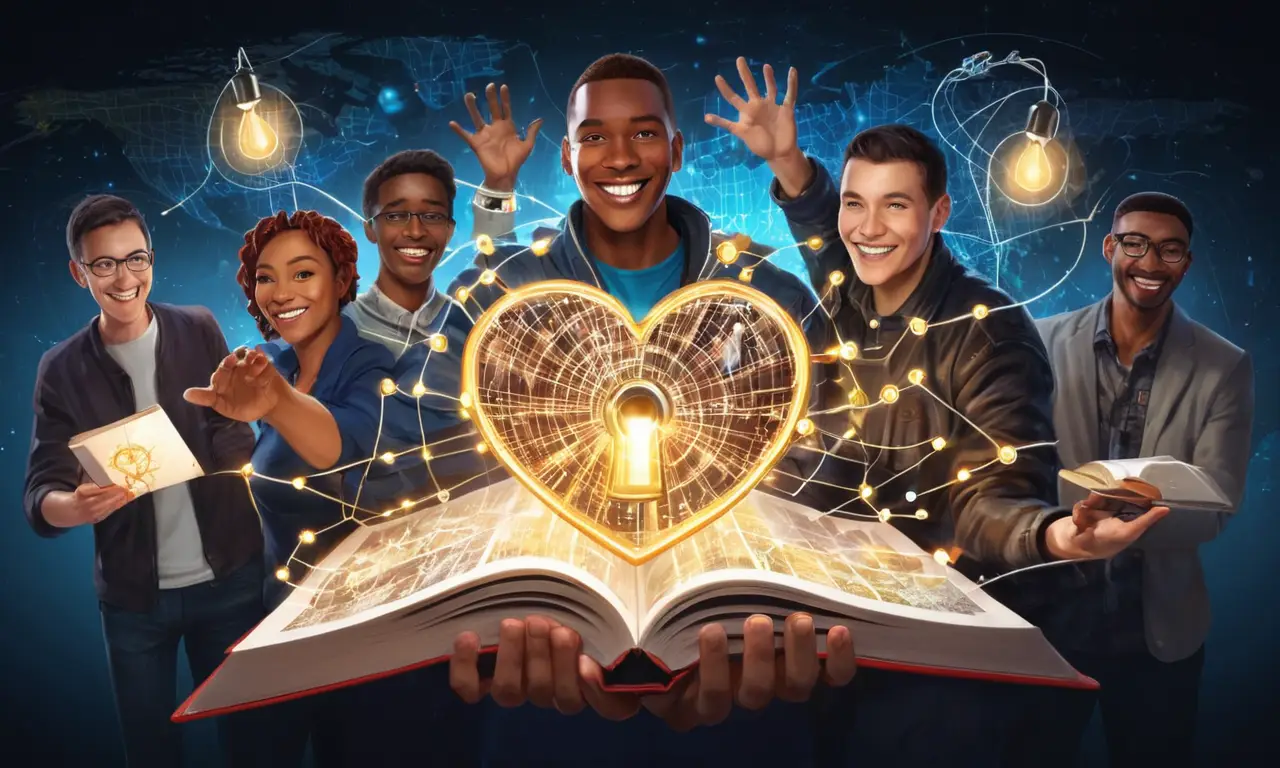
Safety is paramount in any interaction with AI systems. Users should feel confident that they are interacting with reliable and secure technology. Developers must prioritize safety measures throughout the entire AI development lifecycle.
Robustness & Security: AI systems should be designed to withstand attacks, vulnerabilities, and unexpected inputs. This involves rigorous testing, security audits, and ongoing monitoring to ensure system resilience.
Explainability & Transparency: Users should have a clear understanding of how AI systems work and how they arrive at their outputs. Explainable AI (XAI) techniques can help make AI decision-making more transparent and understandable to users.
Human Oversight: While AI can automate many tasks, human oversight remains crucial for critical decisions and situations where ethical considerations are complex.
Respectful Communication
AI systems should engage in communication that is respectful, inclusive, and considerate of diverse perspectives. This requires careful attention to language, tone, and the potential impact of AI-generated content.
Bias Mitigation: AI models can inherit and amplify biases present in the data they are trained on. Developers must actively work to identify and mitigate bias in both training data and AI algorithms to ensure fair and equitable outcomes.
Inclusive Language: AI systems should use language that is inclusive and respectful of all individuals, regardless of their background, identity, or beliefs. This involves avoiding harmful stereotypes, discriminatory language, and offensive content.
Empathy & Understanding: While AI cannot truly feel emotions, it can be designed to recognize and respond to human emotions in a sensitive and empathetic manner.
Avoiding Bias and Discrimination
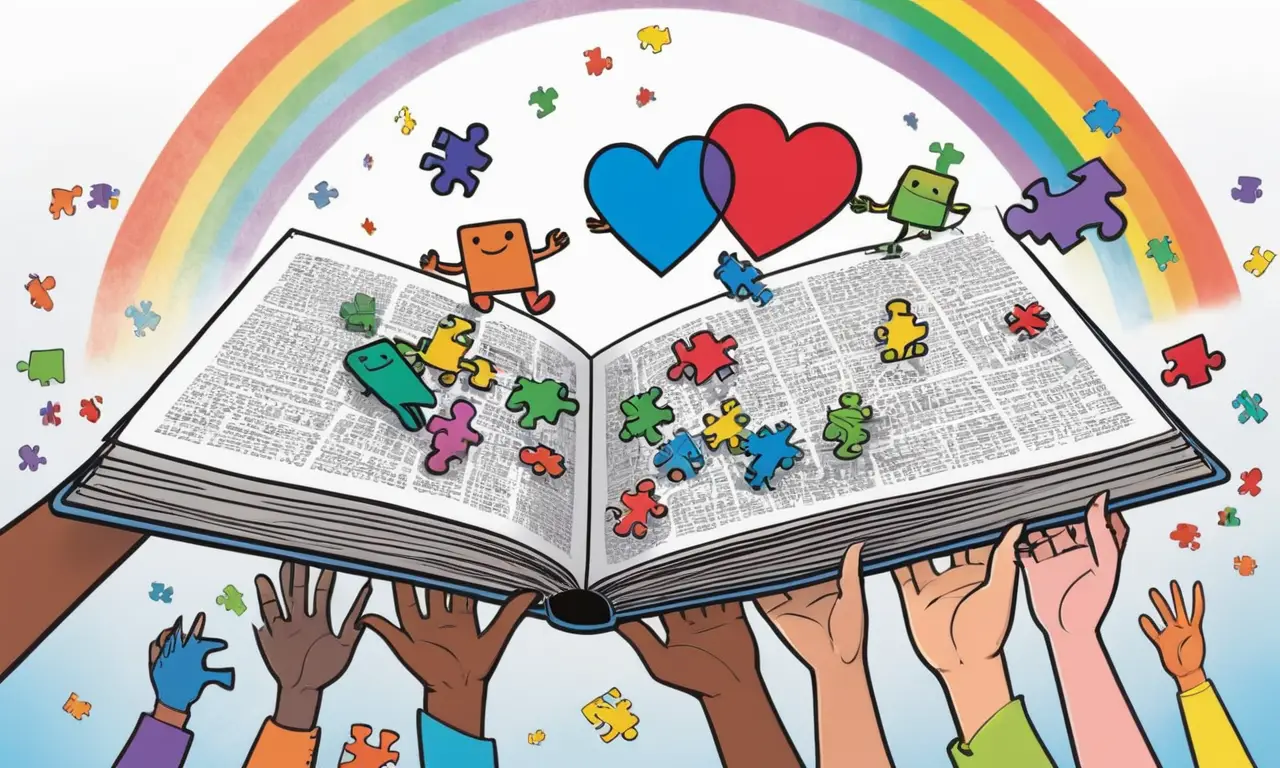
Bias in AI systems can have serious consequences, perpetuating existing inequalities and leading to unfair or discriminatory outcomes. It is essential to proactively address bias throughout the AI development process.
Data Diversity: Training AI models on diverse and representative datasets is crucial for mitigating bias. Data should reflect the diversity of human experiences, perspectives, and backgrounds.
Fairness Metrics: Developers should use fairness metrics to evaluate AI systems for potential bias. These metrics can help identify disparities in outcomes across different demographic groups.
Bias Testing & Auditing: Regular testing and auditing of AI systems can help detect and address bias over time. This involves using diverse test cases and evaluating the performance of AI models across different user groups.
Conclusion
Ethical AI development is an ongoing process that requires continuous reflection, collaboration, and a commitment to upholding human values. By adhering to ethical principles, prioritizing safety and respect in interactions, and actively addressing bias and discrimination, we can harness the transformative power of AI for the benefit of all. It is our collective responsibility to ensure that AI technology serves humanity and contributes to a more just and equitable future.